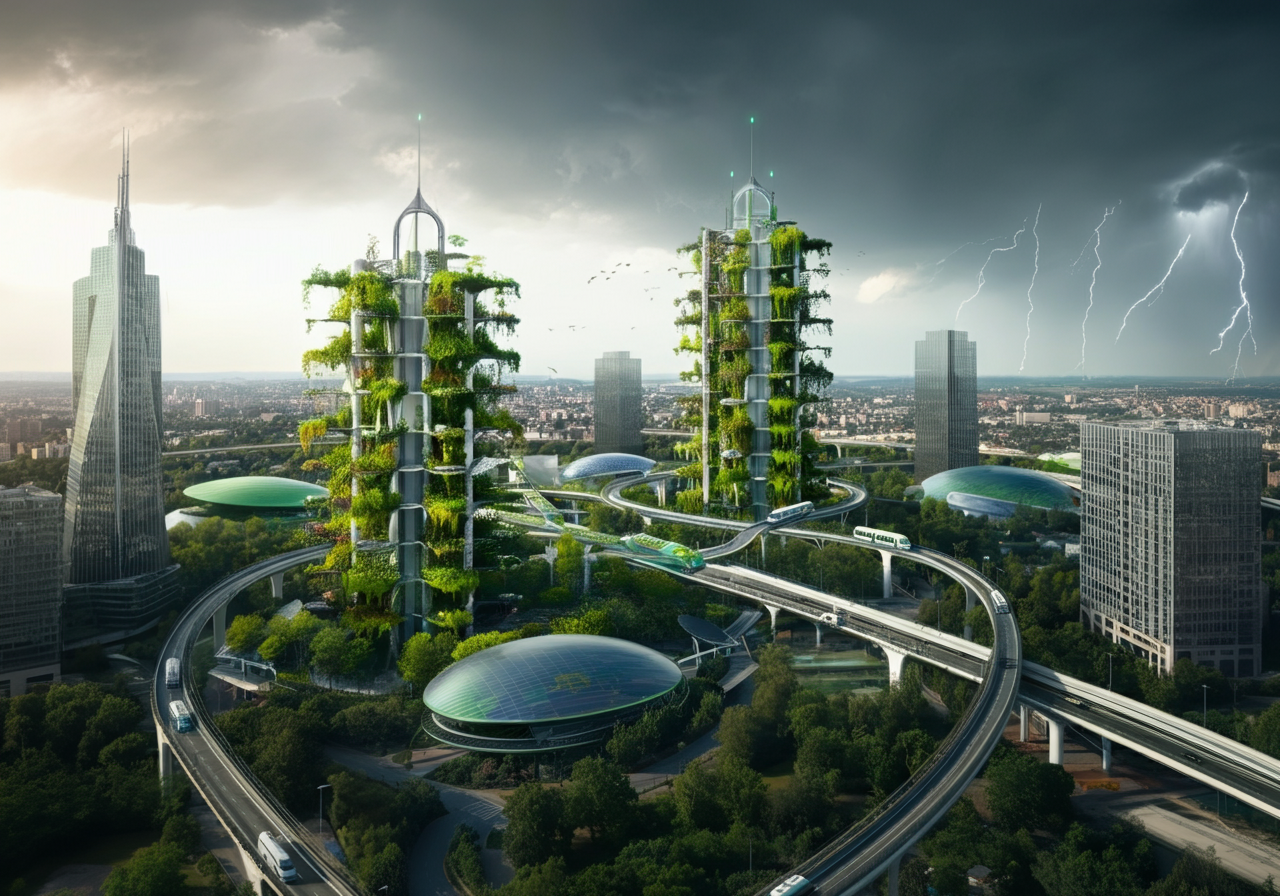
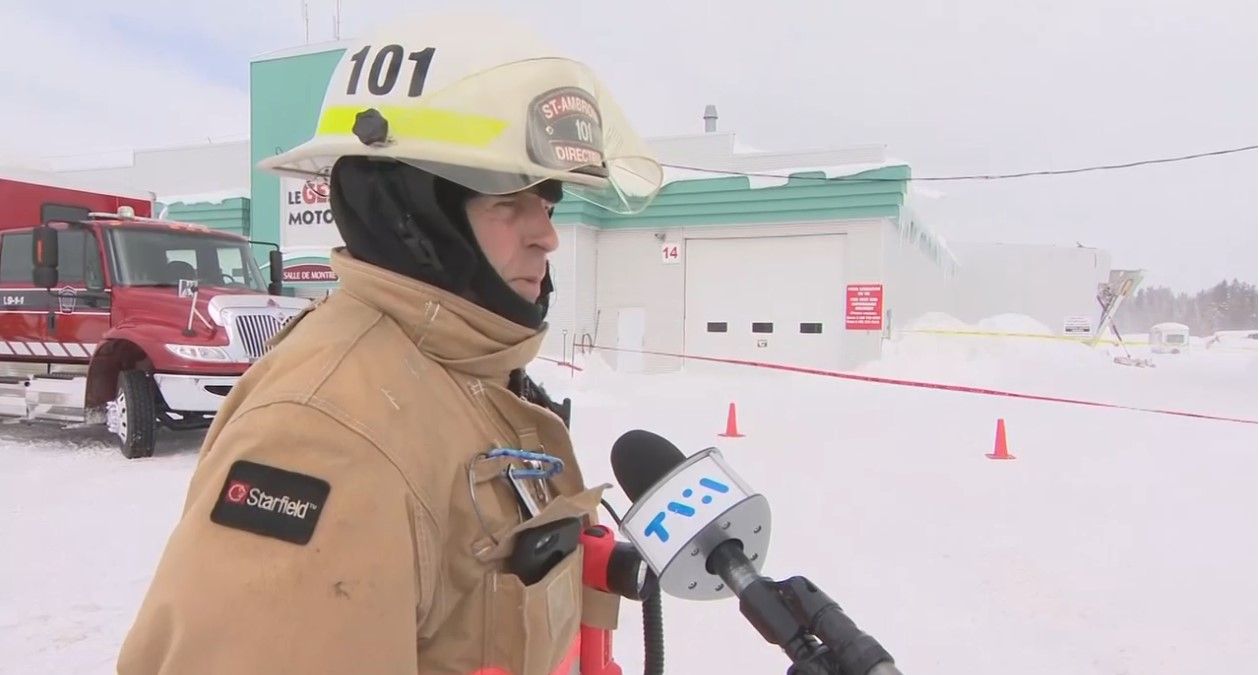
Abstract
This case study examines the efficacy of Domely DeepSnow™ , an AI-powered Software-as-a-Service (SaaS) platform for proactive snow load management. Conducted in collaboration with insurance partners serving clients in Canada, the research analyzes the system's return on investment (ROI) and its impact on customer experience. The analysis compares historical snow-related claims data from 2016 to 2020 with the severity and timing of alerts generated by Domely DeepSnow™. Customer feedback on alert relevance and veracity, gathered through insurer -conducted surveys, is also incorporated. Further, data from academic publications and news reports supplement the analysis by providing insights into claims and events outside the insurance partner's portfolio.
Domely DeepSnow™ utilizes weather data and building characteristics to generate alerts and can be retroactively applied to historical periods. For this study, building weight limits were estimated using National Building Code guidelines, enabling the generation of alerts for years preceding the insurer's adoption of the software.
Results
Economic justification
An actuarial analysis, based on five years of observed roof collapse data, was conducted to determine the probability of collapse in relation to Domely DeepSnow™ 's risk segmentation and alert system. The following table presents the theoretical risk distribution for an insurer during an average winter:
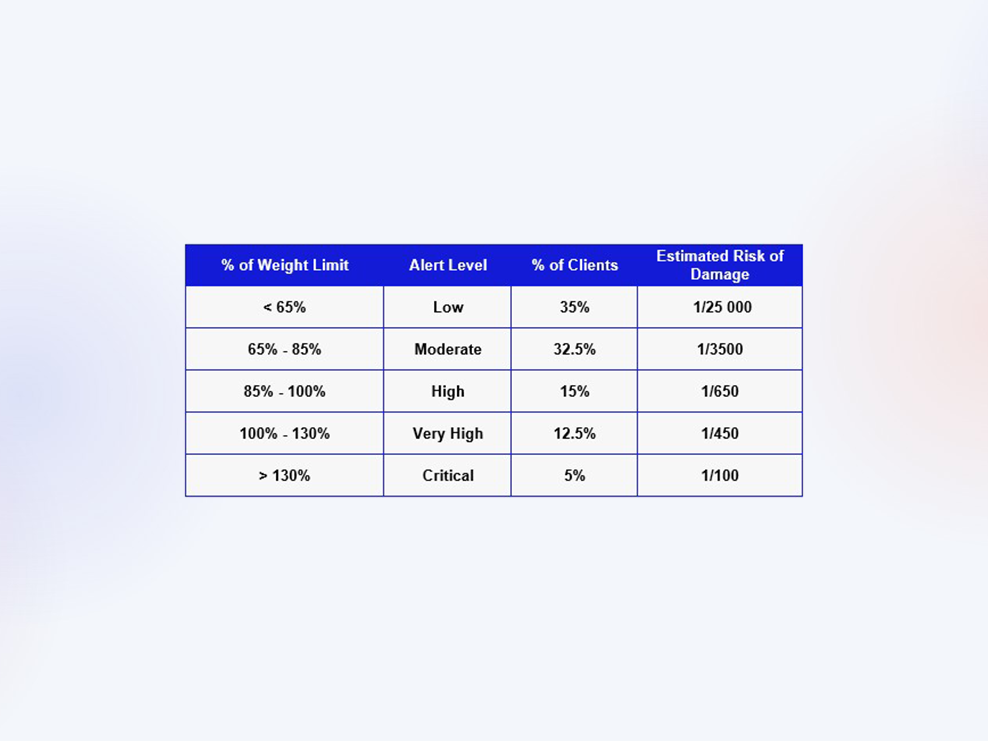
Using a hypothetical portfolio of 15,000 insured buildings and an average collapse cost of $335,000 (Strobel & Liel, 2013) a theoretical cost projection was generated. It's important to acknowledge that these figures are inherently subject to variability due to fluctuations in winter severity and building-specific risk factors.
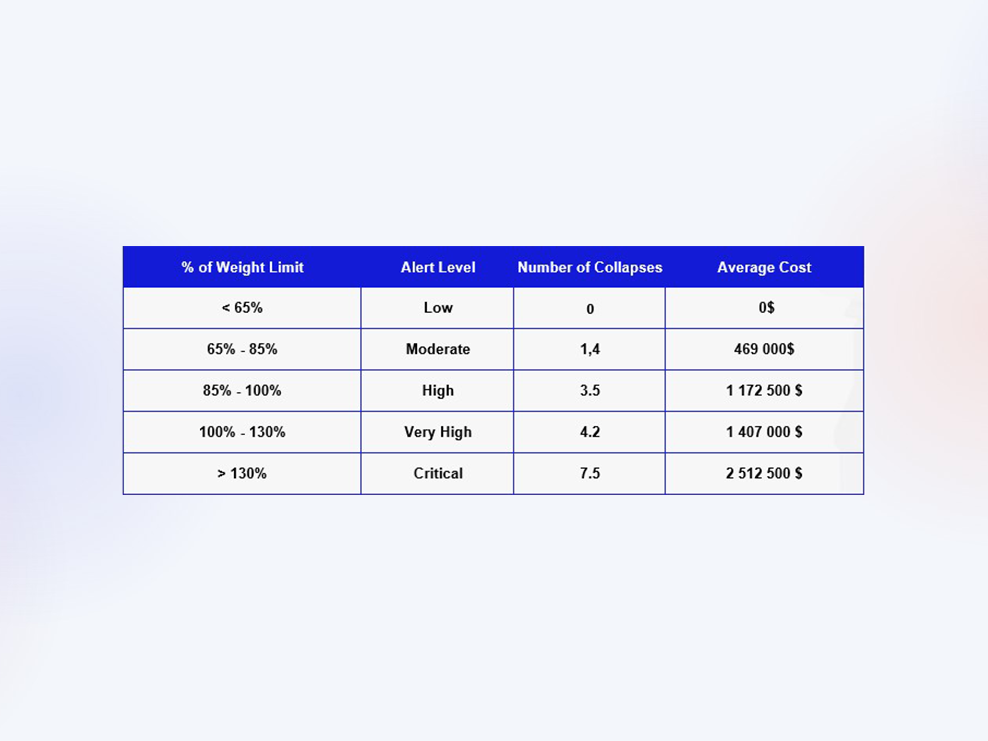
To estimate potential cost savings, we considered various scenarios, assuming that 1 in 5, 10, 20, or 50 alerted clients would take preventative action based on the alert severity. This conservative approach acknowledges that alert relevance directly influences action, with higher severity alerts demonstrating increased engagement, as evidenced by higher open rates for targeted communications.
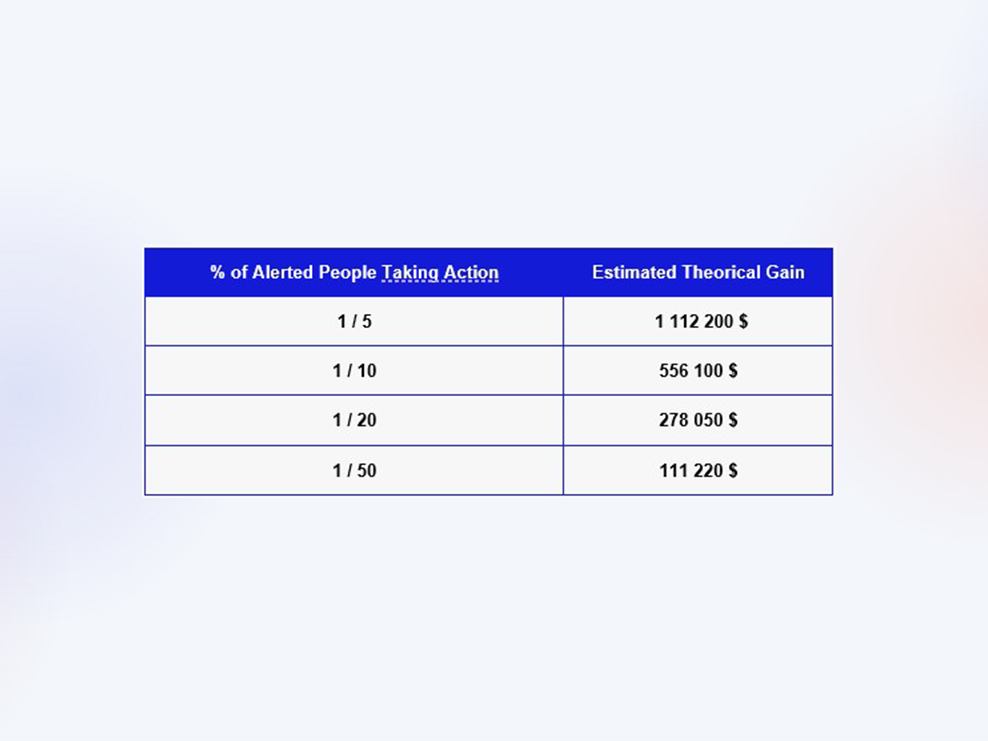
Given that the cost of monitoring 15,000 buildings using Domely DeepSnow™ is under $100,000, a positive ROI for the initiative is clearly demonstrable.
Moreover, analysis of Canadian insurance data¹ from commercial buildings reveals minor roof collapses occur at a rate of 0.7%, with an average claim amount of $40,000, while major roof collapses, though less frequent at 0.04%, result in an average claim of $200,000. This translates to an average risk exposure of $360 per building per winter season, once again demonstrating a positive ROI.
From the point of view of the building owner or manager, the cost of assessing the snow level on the roof, A task performed automatically and remotely by Domely DeepSnow™ , is also a factor for positive ROI. The average price reported for a in-person professional snow level assessment is $781, including travel, time and a detailed report².
Enhanced Customer Experience
Beyond financial gains, Domely DeepSnow™ fosters a proactive and customer-centric approach, aligning with the strategic priorities of insurers and property managers. Preventing claims, inherently, provides the optimal customer experience.
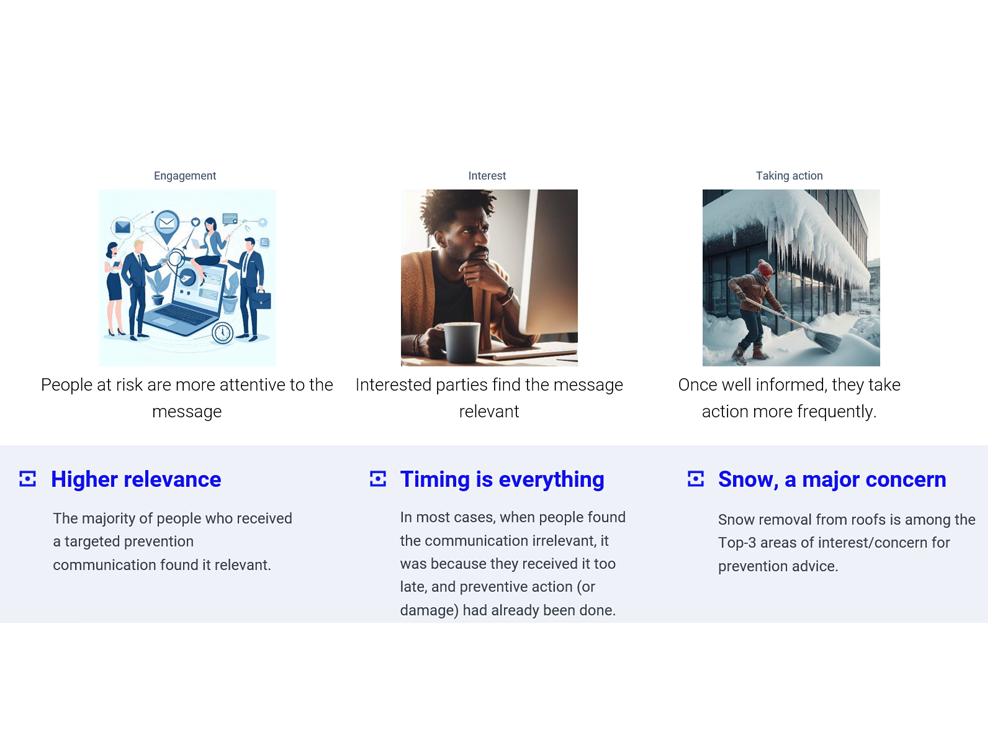
Survey data revealed a high level of satisfaction with targeted prevention communications, with over 70% of recipients finding the information relevant. A significant portion of those who considered the communication irrelevant (30%) had already taken preventative action, indicating an existing awareness of potential risks. Furthermore, snow removal from roofs consistently ranked among the top three concerns, highlighting its importance for building owners and validating the system's focus on this critical area.
The ability to avert potential catastrophes for both insurers and their clients carries immeasurable value. The ramifications of roof collapse often extend beyond property damage, leading to evacuations (reported in 28% of cases) and business interruptions (57% of cases). The average closure duration following a snow-related collapse was 122 days, or just over four months (Geis, Strobel, & Liel, 2012)
Tragically, snow-related collapses also contribute to injuries and fatalities annually. In 2006, a series of major collapses worldwide resulted in over 130 deaths (ALMahdi & al, 2020; Strasser, 2008; Geis, Strobel, & Liel, 2012). While less frequently impacted, residential buildings are not immune. In 2009, a major snowstorm in China led to the collapse of 1,200 homes and damage to 1,900 others, incurring over $497 million in damages (Clem, 2009). Even public buildings are vulnerable, as exemplified by the roof collapses of four schools in the Boston and New Hampshire region during the 2014-2015 winter season (ALMahdi & al, 2020).
A Success Story
Definitive predictions about averted damages are challenging. However, a compelling anecdote emerges from a 2022 incident³ where a building with characteristics similar to one of our client's properties collapsed under snow load.
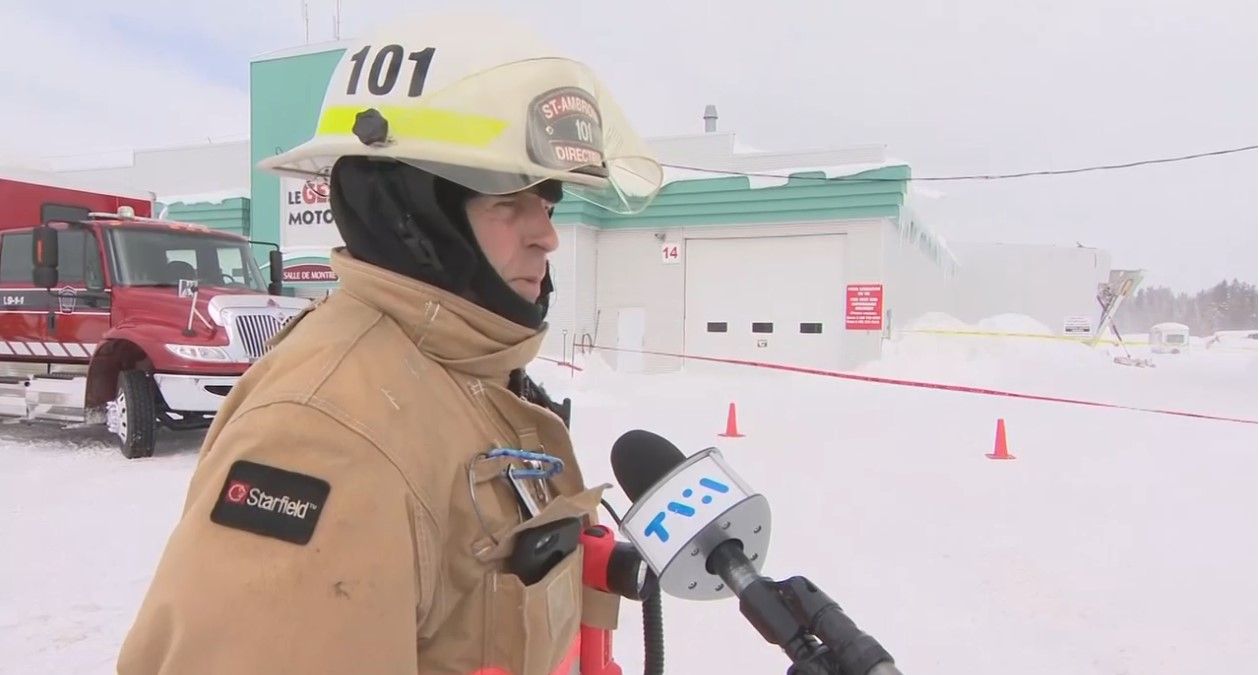
Domely DeepSnow™ provided our client with weeks of advance notice, predicting a critical load exceeding 170%. This early warning enabled preemptive action, including snow removal , averting a potential $573,000 loss (excluding damaged goods and reconstruction costs, and based on an estimated 25% building collapse).
Domely DeepSnow™ demonstrates a strong economic rationale by showcasing a positive ROI through potential claim cost reduction. Moreover, it cultivates a customer-centric approach by fostering proactive communication and mitigating the risk of catastrophic events, ultimately enhancing customer experience. The system's success is further evidenced by a case study demonstrating its effectiveness in preventing a substantial financial loss.
- Data from Canadian insurance partners
- Data from roofing industry partners
- https://www.journaldequebec.com/2022/03/08/le-toit-dun-concessionnaire-de-saint-ambroise-seffondre-1
- Strobel, K., & Liel, A. B. (2013). Snow load damage to buildings: Physical and economic impacts. Proceedings of the Institution of Civil Engineers - Forensic Engineering 166(3), (pp. 116-133).
- Geis, J., Strobel, K., & Liel, A. (2012). Snow-induced building failures. Journal of Performance of Constructed Facilities, 26(4), 377–388.
- ALMahdi, F., & al. (2020). Investigation of snow-induced collapse in bozüyük market. Engineering Failure Analysis, 118, 1-11. ; Strasser, U. (2008). Snow loads in a changing climate: new risks? Natural Hazards and Earth System Sciences, 8(1), 1-8
- Clem, W. (2009, Nov 13). Wen visits Hebei after disastrous snowfall; Cloud seeding may be to blame for chaos. p. 7
Read more in this category
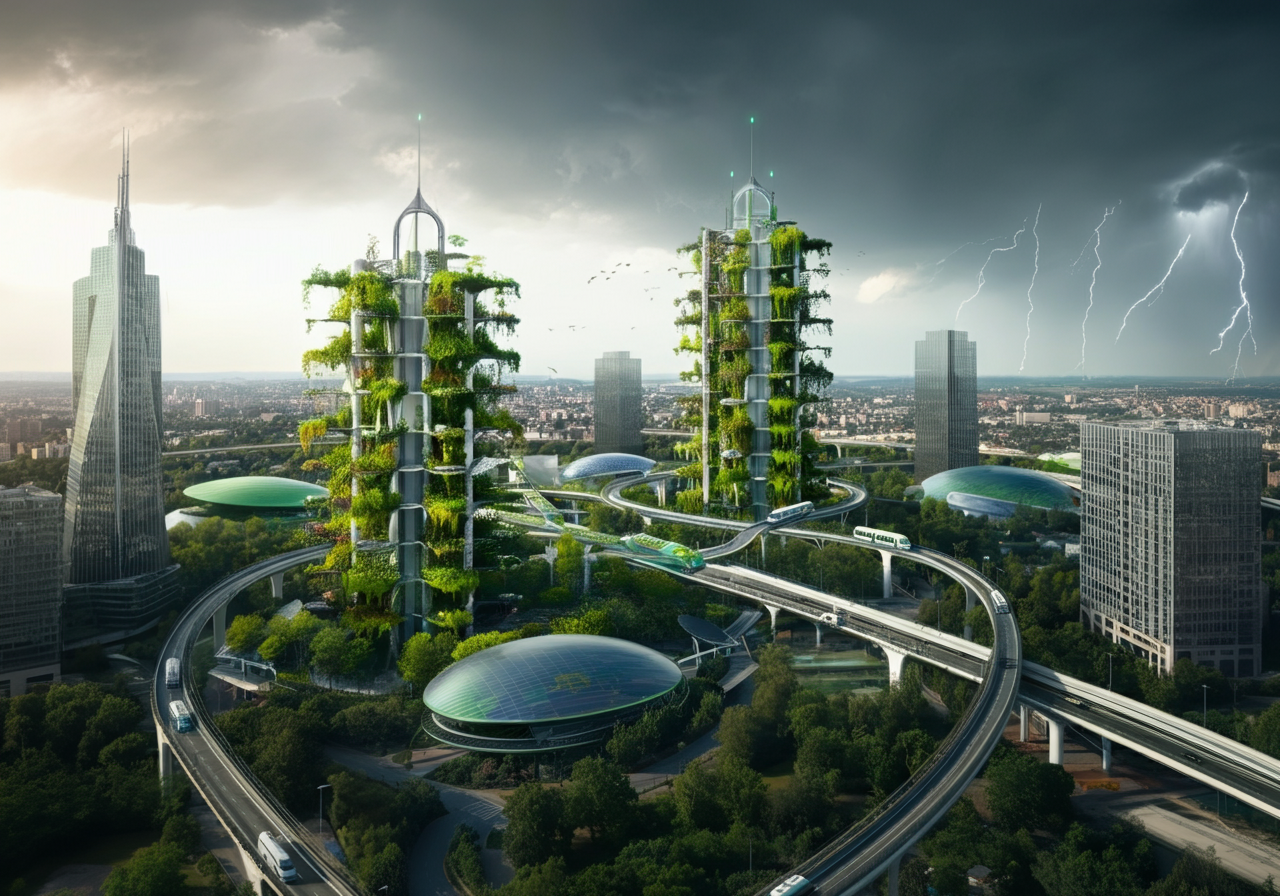
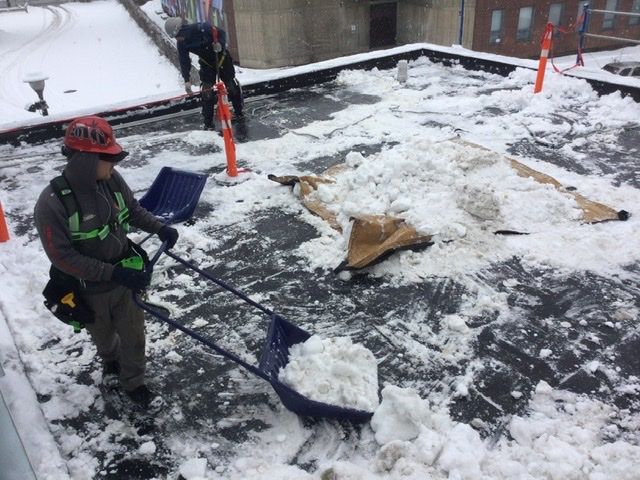
Safeguarding Workers in Winter - A Comprehensive Guide to Roof Snow Removal Safety
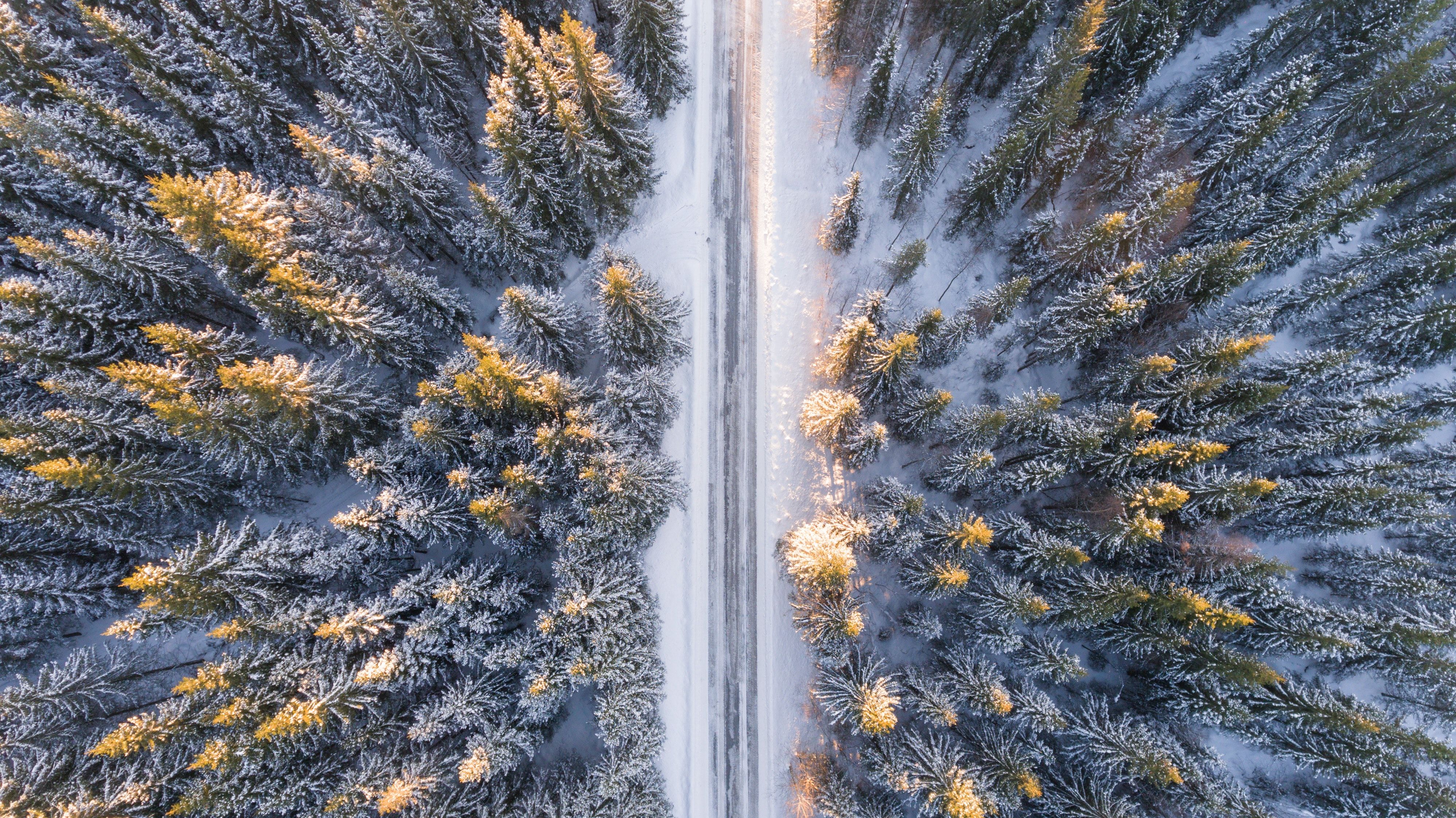
Climate Change is Changing the Rules - Why You Need a New Approach to Roof Snow Load
Get a quote
Want a quote or have questions? Would you like to better understand how our smart offering and unique data can help you?